Consistent Hashing
In this tutorial, we are going to discuss about consistent hashing, another essential system design concept. This tutorial discusses key concepts of consistent hashing, which come in handy while scaling out the distributed storage systems. This idea is frequently applied in designing various real-time applications and solving system design questions during interviews.
The act of distributing data across a set of nodes is called data partitioning. There are two challenges when we try to distribute data.
- How do we know on which node a particular piece of data will be stored?
- When we add or remove nodes, how do we know what data will be moved from existing nodes to the new nodes? Additionally, how can we minimize data movement when nodes join or leave?
A naive approach will use a suitable hash function that maps the data key to a number. Then, find the server by applying modulo on this number and the total number of servers. For example:

The scheme described in the above diagram solves the problem of finding a server for storing/retrieving the data. But when we add or remove a server, we have to remap all the keys and move our data based on the new server count, which will be a complete mess!
Use the Consistent Hashing algorithm to distribute data across nodes. Consistent Hashing maps data to physical nodes and ensures that only a small set of keys move when servers are added or removed.
What is Consistent Hashing?
Before diving deep into Consistent Hashing, let’s first understand what hashing is?
Hashing is a technique used to efficiently retrieve information and improve the performance of insert, delete, and search operations. It involves using a hash function to map data to a storage pool based on key values. For example, we can generate a random number and use it to map data to a server by taking the modulo of the total number of servers. However, this approach is only effective when the number of servers are fixed.
In distributed systems, the number and location of servers may change, making it necessary to have a more efficient way of handling and organizing requests for a scalable application. Consistent hashing is an improvement over normal hashing that addresses these issues.
In consistent hashing, user and server locations are virtually represented in a circular structure called a hash ring. The hash ring is considered infinite and can accommodate any number of servers, which are assigned to random locations based on a hash function. This allows for flexible allocation of servers and efficient handling of requests in a distributed system.
Consistent Hashing technique stores the data managed by a distributed system in a ring. Each node in the ring is assigned a range of data. Here is an example of the consistent hash ring:

With consistent hashing, the ring is divided into smaller, predefined ranges. Each node is assigned one of these ranges. The start of the range is called a token. This means that each node will be assigned one token. The range assigned to each node is computed as follows:
Range start: Token value
Range end: Next token value – 1
Here are the tokens and data ranges of the four nodes described in the above diagram:
Server | Token | Range Start | Range End |
Server 1 | 1 | 1 | 25 |
Server 2 | 26 | 26 | 50 |
Server 3 | 51 | 51 | 75 |
Server 4 | 76 | 76 | 100 |
Whenever the system needs to read or write data, the first step it performs is to apply the MD5 hashing algorithm to the key. The output of this hashing algorithm determines within which range the data lies and hence, on which node the data will be stored. As we saw above, each node is supposed to store data for a fixed range. Thus, the hash generated from the key tells us the node where the data will be stored.
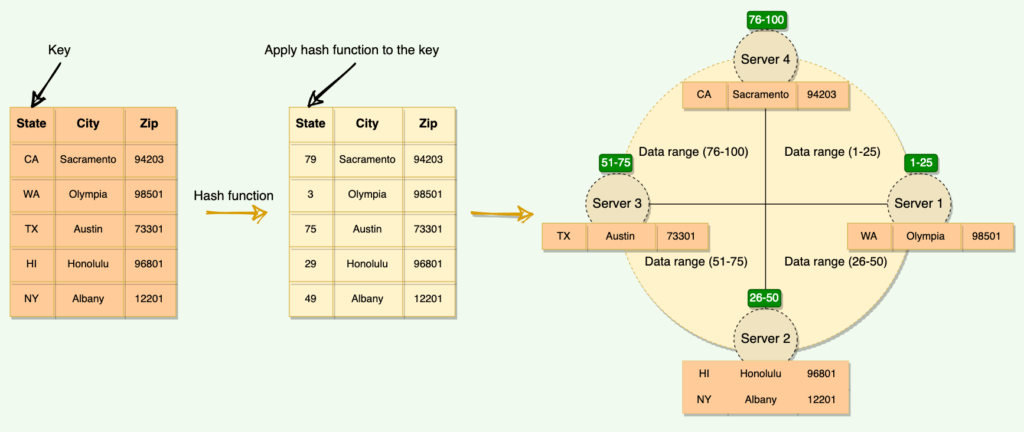
The Consistent Hashing scheme described above works great when a node is added or removed from the ring, as in these cases, since only the next node is affected. For example, when a node is removed, the next node becomes responsible for all of the keys stored on the outgoing node. However, this scheme can result in non-uniform data and load distribution. This problem can be solved with the help of Virtual nodes.
Why do we need Consistent Hashing?
The traditional hashing method is not well-suited for use in distributed systems where multiple users are requesting services from multiple servers. This is because it assumes that the number of servers and their mapping locations are fixed, which is not the case in a distributed system. If a server fails or goes offline, the traditional method requires significant computation to remap requests to different servers, which can affect the throughput and latency of the service.
In distributed systems, multiple nodes interact with each other and the number of nodes can change due to changes in traffic or maintenance. For example, if we have five nodes in the system and there is an increase in traffic, we may need to add two more nodes to the system, bringing the total to seven. Using traditional hashing, we would need to recompute the mapping of requests, as the hash was previously based on five nodes but now we have seven. Similarly, if the number of nodes decreases due to maintenance or failure, we would need to recompute the mapping again. This is inefficient and requires a lot of redundant computation and data reshuffling within the cluster.
In situations where the number of operational servers is not fixed, the traditional hashing method becomes increasingly inefficient as the number of servers increases, since it requires more and more computation and reassignment of requests. To address these shortcomings, the concept of consistent hashing was developed as a more dynamic solution.
Virtual nodes
Adding and removing nodes in any distributed system is quite common. Existing nodes can die and may need to be decommissioned. Similarly, new nodes may be added to an existing cluster to meet growing demands. To efficiently handle these scenarios, Consistent Hashing makes use of virtual nodes (or Vnodes).
As we saw above, the basic Consistent Hashing algorithm assigns a single token (or a consecutive hash range) to each physical node. This was a static division of ranges that requires calculating tokens based on a given number of nodes. This scheme made adding or replacing a node an expensive operation, as, in this case, we would like to rebalance and distribute the data to all other nodes, resulting in moving a lot of data. Here are a few potential issues associated with a manual and fixed division of the ranges:
- Adding or removing nodes: Adding or removing nodes will result in recomputing the tokens causing a significant administrative overhead for a large cluster.
- Hotspots: Since each node is assigned one large range, if the data is not evenly distributed, some nodes can become hotspots.
- Node rebuilding: Since each node’s data might be replicated (for fault-tolerance) on a fixed number of other nodes, when we need to rebuild a node, only its replica nodes can provide the data. This puts a lot of pressure on the replica nodes and can lead to service degradation.
To handle these issues, Consistent Hashing introduces a new scheme of distributing the tokens to physical nodes. Instead of assigning a single token to a node, the hash range is divided into multiple smaller ranges, and each physical node is assigned several of these smaller ranges. Each of these subranges is considered a Vnode. With Vnodes, instead of a node being responsible for just one token, it is responsible for many tokens (or subranges).
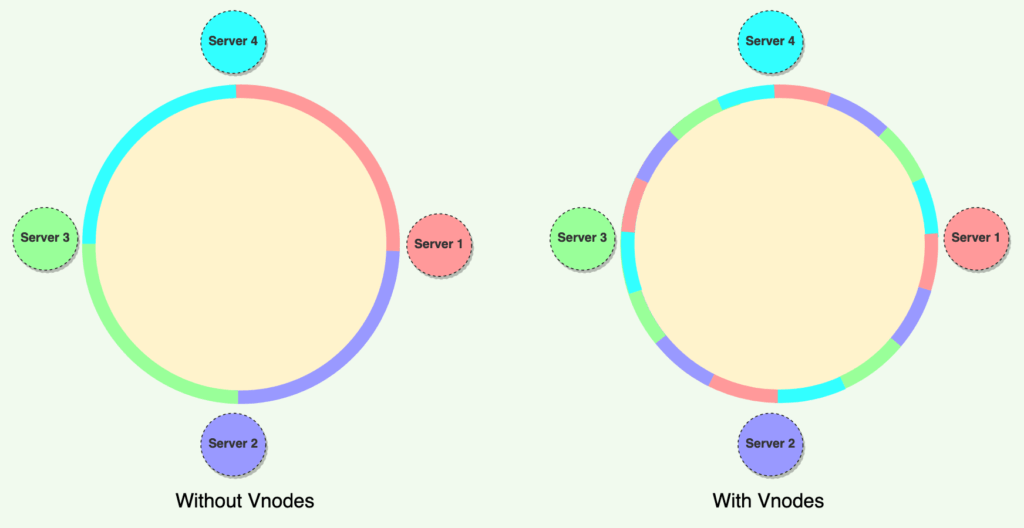
Practically, Vnodes are randomly distributed across the cluster and are generally non-contiguous so that no two neighboring Vnodes are assigned to the same physical node or rack. Additionally, nodes do carry replicas of other nodes for fault tolerance. Also, since there can be heterogeneous machines in the clusters, some servers might hold more Vnodes than others. The figure below shows how physical nodes A, B, C, D, & E use Vnodes of the Consistent Hash ring. Each physical node is assigned a set of Vnodes and each Vnode is replicated once.
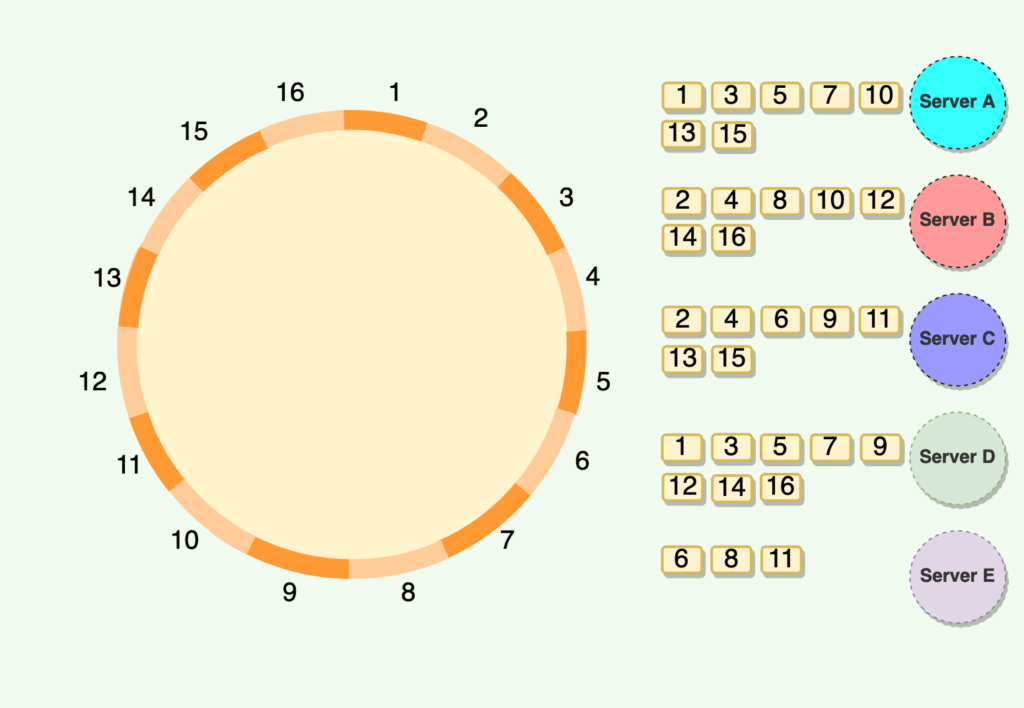
Advantages of Vnodes
Vnodes gives the following advantages:
- As Vnodes help spread the load more evenly across the physical nodes on the cluster by dividing the hash ranges into smaller subranges, this speeds up the rebalancing process after adding or removing nodes. When a new node is added, it receives many Vnodes from the existing nodes to maintain a balanced cluster. Similarly, when a node needs to be rebuilt, instead of getting data from a fixed number of replicas, many nodes participate in the rebuild process.
- Vnodes make it easier to maintain a cluster containing heterogeneous machines. This means, with Vnodes, we can assign a high number of sub-ranges to a powerful server and a lower number of sub-ranges to a less powerful server.
- In contrast to one big range, since Vnodes help assign smaller ranges to each physical node, this decreases the probability of hotspots.
That’s all about Consistent Hashing in system design. If you have any queries or feedback, please write us at contact@waytoeasylearn.com. Enjoy learning, Enjoy system design..!!