MapReduce Detailed Overview

Map Reduce – Key-Value Pair
What is a key-value pair in Hadoop?
Apache Hadoop is used mainly for Data Analysis. We look at statistical and logical techniques in data Analysis to describe, illustrate and evaluate data. Hadoop deals with structured, unstructured and semi-structured data. In Hadoop, when the schema is static we can directly work on the column instead of keys and values, but, when the schema is not static, then we will work on keys and values. Keys and values are not the intrinsic properties of the data, but they are chosen by user analyzing the data.
MapReduce is the core component of Hadoop which provides data processing. Hadoop MapReduce is a software framework for easily writing an application that processes the vast amount of structured and unstructured data stored in the Hadoop Distributed File System (HDFS). MapReduce works by breaking the processing into two phases: Map phase and Reduce phase. Each phase has key-value as input and output.
Key-value pair Generation in MapReduce
In MapReduce process, before passing the data to the mapper, data should be first converted into key-value pairs as mapper only understands key-value pairs of data. Key-value pairs in Hadoop MapReduce is generated as follows:
InputSplit – It is the logical representation of data. The data to be processed by an individual Mapper is presented by the InputSplit.
RecordReader – It communicates with the InputSplit and it converts the Split into records which are in form of key-value pairs that are suitable for reading by the mapper. By default, RecordReader uses TextInputFormat for converting data into a key-value pair. RecordReader communicates with the InputSplit until the file reading is not completed.
In MapReduce, map function processes a certain key-value pair and emits a certain number of key-value pairs and the Reduce function processes values grouped by the same key and emits another set of key-value pairs as output. The output types of the Map should match the input types of the Reduce as shown below
Map: (K1, V1) -> list (K2, V2)
Reduce: {(K2, list (V2 }) -> list (K3, V3)Â
On what basis is a key-value pair generated in Hadoop?
Generation of a key-value pair in Hadoop depends on the data set and the required output. In general, the key-value pair is specified in 4 places: Map input, Map output, Reduce input and Reduce output.
1. Map Input
Map-input by default will take the line offset as the key and the content of the line will be the value as Text. By using custom InputFormat we can modify them.
2. Map Output
Map basic responsibility is to filter the data and provide the environment for grouping of data based on the key.
Key – It will be the field/ text/ object on which the data has to be grouped and aggregated on the reducer side.
Value – It will be the field/ text/ object which is to be handled by each individual reduce method.
3. Reduce Input
The output of Map is the input for reduce, so it is same as Map-Output.
4. Reduce Output
It depends on the required output.
MapReduce key-value pair Example
Suppose, the content of the file which is stored in HDFS is My name is Ashok Kumar. Using InputFormat, we will define how this file will split and read. By default, RecordReader uses TextInputFormat to convert this file into a key-value pair.
Key – It is offset of the beginning of the line within the file.
Value – It is the content of the line, excluding line terminators.
From the above content of the file-
Key is 0
Value is My name is Ashok Kumar.
Autonomy of MapReduce

- In MapReduce, chunks are processed in isolation by tasks called Mappers.
- The outputs from the mappers ae denoted as intermediate outputs.
- The process of bringing together intermediate outputs into a set of Reducers is known as Shuffling and sort process.
- Intermediate outputs will be given as input into second set of tasks called Reducers.
- The Reducers produce the final outputs.
- Overall, MapReduce breaks the data flow into 2 phases, map phase and reduce phase.

MapReduce closer lookÂ

Input FilesÂ
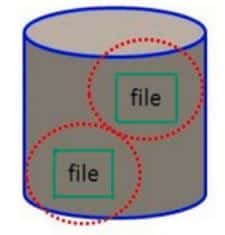
- Input files are where the data for a MapReduce task is initially stored.
- The input files typically reside in a distributed file system (e.g HDFS)
- The format of input files is arbitrary
- Line-based log files
- Binary files
- Multi-line input records
- Or something else entirely