InputFormat
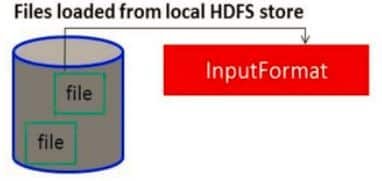
Hadoop can process many different types of data formats, from flat text files to databases. Hadoop InputFormat checks the Input-Specification of the job. InputFormat split the Input file into InputSplit and assign to individual Mapper. How the input files are split up and read in Hadoop is defined by the InputFormat.
An Hadoop InputFormat is the first component in Map-Reduce, it is responsible for creating the input splits and dividing them into records. Initially, the data for a MapReduce task is stored in input files, and input files typically reside in HDFS. Although these files format is arbitrary, line-based log files and binary format can be used. Using InputFormat we define how these input files are split and read.
The InputFormat class is one of the fundamental classes in the Hadoop MapReduce framework which provides the following functionality:
- The files or other objects that should be used for input is selected by the InputFormat.
- InputFormat defines the Data splits, which defines both the size of individual Map tasks and its potential execution server.
- InputFormat defines the RecordReader, which is responsible for reading actual records from the input files.
How we get the data to mapper?
We have 2 methods to get the data to mapper in MapReduce: getsplits() and createRecordReader() as shown below
public abstract class InputFormat<K, V> { public abstract List<InputSplit> getSplits(JobContext context) throws IOException, InterruptedException; public abstract RecordReader<K, V> createRecordReader(InputSplit split, TaskAttemptContext context) throws IOException, InterruptedException; }
Types of InputFormat in MapReduce
1. FileInputFormat
FileInputFormat is the base class for all implementations of InputFormat that use files as their data source (See following figure).
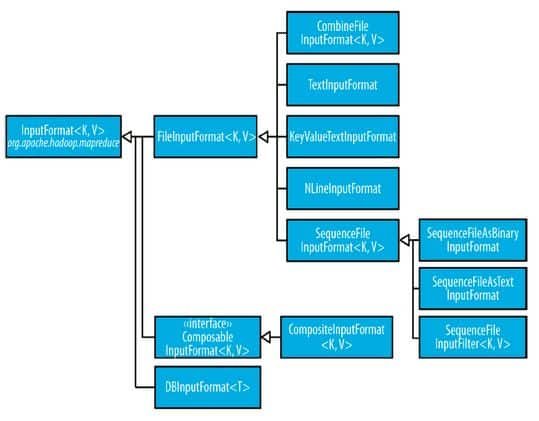
FileInputFormat provides 2 things: a place to define which files are included as the input to a job, and an implementation for generating splits for the input files.
Input paths of FileInputFormat
The input to a job is specified as a collection of paths, which offers great flexibility in constraining the input. FileInputFormat offers four static convenience methods for setting a Job’s input paths
public static void addInputPath(Job job, Path path) public static void addInputPaths(Job job, String commaSeparatedPaths) public static void setInputPaths(Job job, Path... inputPaths) public static void setInputPaths(Job job, String commaSeparatedPaths)
- The addInputPath() and addInputPaths() methods add a path or paths to the list of inputs.
- The setInputPaths() methods set the entire list of paths in one go.
A path may represent a file, a directory, or, by using a glob, a collection of files and directories. A path representing a directory includes all the files in the directory as input to the job. The add and set methods allow files to be specified by inclusion only. To exclude certain files from the input, you can set a filter using the setInputPathFilter() method on FileInputFormat. Even if you don’t set a filter, FileInputFormat uses a default filter that excludes hidden files (those whose names begin with a dot or an underscore). If you set a filter by calling setInputPathFilter(), it acts in addition to the default filter. In other words, only non hidden files that are accepted by your filter get through.
FileInputFormat input splits
Given a set of files, how does FileInputFormat turn them into splits?
FileInputFormat splits only large files—here, “large” means larger than an HDFS block. The split size is normally the size of an HDFS block, which is appropriate for most applications; however, it is possible to control this value by setting various Hadoop properties, as shown in below table.

Small files and CombineFileInputFormat
Hadoop works better with a small number of large files than a large number of small files. One reason for this is that FileInputFormat generates splits in such a way that each split is all or part of a single file. If the file is very small (“small” means significantly smaller than an HDFS block) and there are a lot of them, each map task will process very little input, and there will be a lot of them (one per file), each of which imposes extra bookkeeping overhead. Compare a 1 GB file broken into eight 128 MB blocks with 10,000 or so 100 KB files. The 10,000 files use one map each, and the job time can be tens or hundreds of times slower than the equivalent one with a single input file and eight map tasks.
The situation is alleviated somewhat by CombineFileInputFormat, which was designed to work well with small files. Where FileInputFormat creates a split per file, CombineFileInputFormat packs many files into each split so that each mapper has more to process. Crucially, CombineFileInputFormat takes node and rack locality into account when deciding which blocks to place in the same split, so it does not compromise the speed at which it can process the input in a typical MapReduce job.
Of course, if possible, it is still a good idea to avoid the many small files case, because MapReduce works best when it can operate at the transfer rate of the disks in the cluster, and processing many small files increases the number of seeks that are needed to run a job. Also, storing large numbers of small files in HDFS is wasteful of the NameNode’s memory.
CombineFileInputFormat isn’t just good for small files. It can bring benefits when processing large files, too, since it will generate one split per node, which may be made up of multiple blocks. Essentially, CombineFileInputFormat decouples the amount of data that a mapper consumes from the block size of the files in HDFS.
Preventing splitting Some applications don’t want files to be split, as this allows a single mapper to process each input file in its entirety. For example, a simple way to check if all the records in a file are sorted is to go through the records in order, checking whether each record is not less than the preceding one. Implemented as a map task, this algorithm will work only if one map processes the whole file.
There are a couple of ways to ensure that an existing file is not split. The first (quickand- dirty) way is to increase the minimum split size to be larger than the largest file in your system. Setting it to its maximum value, Long.MAX_VALUE, has this effect. The second is to subclass the concrete subclass of FileInputFormat that you want to use, to override the isSplitable() method3 to return false. For example, here’s a nonsplittable TextInputFormat
import org.apache.hadoop.fs.Path; import org.apache.hadoop.mapreduce.JobContext; import org.apache.hadoop.mapreduce.lib.input.TextInputFormat; /** * * @author ashok.mariyala * */ public class NonSplittableTextInputFormat extends TextInputFormat { @Override protected boolean isSplitable(JobContext context, Path file) { return false; } }
File information in the mapper
A mapper processing a file input split can find information about the split by calling the getInputSplit() method on the Mapper’s Context object. When the input format derives from FileInputFormat, the InputSplit returned by this method can be cast to a FileSplit to access the file information listed below,

Text Input
Hadoop excels at processing unstructured text.
1. TextInputFormat
TextInputFormat is the default InputFormat of MapReduce. The TextInputFormat works as an InputFormat for plain text files. Files are broken into lines. Each record is a line of input.
Key: A LongWritable, is the byte offset within the file of the beginning of the line.
Value: The value is the contents of the line, excluding any line terminators (e.g., newline or carriage return), and is packaged as a Text object.
So, a file containing the following text
I'm Ashok Kumar, Java Developer from Hyderabad, India. I have a Master Degree in Computer Applications from S.R.K.R Engineering College, Bhimavaram. I achieved Gold Medal in my Master Degree.
is divided into one split of four records. The records are interpreted as the following key-value pairs
(0, I'm Ashok Kumar, Java Developer from Hyderabad, India.) (56, I have a Master Degree in Computer Applications from S.R.K.R Engineering College, Bhimavaram.) (150, I achieved Gold Medal in my Master Degree.)
2. KeyValueTextInputFormat
KeyValueTextInputFormat is similar to TextInputFormat as it also treats each line of input as a separate record. While TextInputFormat treats entire line as the value, but the KeyValueTextInputFormat breaks the line itself into key and value by a tab character (‘/t’). Here Key is everything up to the tab character while the value is the remaining part of the line after tab character.
You can specify the separator via the mapreduce.input.keyvalueline recordreader.key.value.separator property. It is a tab character by default. Consider the following input file, where ? represents a (horizontal) tab character.
line1 → Hi this is Ashok. line2 → I am from Hyderabad. line3 → I did master of computer applications. line4 → I am a Java software developer.
Like in the TextInputFormat case, the input is in a single split comprising four records, although this time the keys are the Text sequences before the tab in each line:
(line1, Hi this is Ashok.) (line2, I am from Hyderabad.) (line3, I did master of computer applications,) (line4, I am a Java software developer.)
NLineInputFormat
With TextInputFormat and KeyValueTextInputFormat, each mapper receives a variable number of lines of input. The number depends on the size of the split and the length of the lines. If you want your mappers to receive a fixed number of lines of input, then NLineInputFormat is the InputFormat to use. Like with TextInputFormat, the keys are the byte offsets within the file and the values are the lines themselves.
N refers to the number of lines of input that each mapper receives. With N set to 1 (the default), each mapper receives exactly one line of input. The mapreduce.input.line inputformat.linespermap property controls the value of N. By way of example, consider these four lines again
I'm Ashok Kumar, Java Developer from Hyderabad, India. I have a Master Degree in Computer Applications from S.R.K.R Engineering College, Bhimavaram. I achieved Gold Medal in my Master Degree. I am passionate to learn the new Technologies.
If, for example, N is 2, then each split contains two lines. One mapper will receive the first two key-value pairs
(0, I'm Ashok Kumar, Java Developer from Hyderabad, India.) (56, I have a Master Degree in Computer Applications from S.R.K.R Engineering College, Bhimavaram.)
and another mapper will receive the second two key-value pairs
(150, I achieved Gold Medal in my Master Degree.) (193, I am passionate to learn the new Technologies.)
The keys and values are the same as those that TextInputFormat produces. The difference is in the way the splits are constructed.
Usually, having a map task for a small number of lines of input is inefficient (due to the overhead in task setup), but there are applications that take a small amount of input data and run an extensive (i.e., CPU-intensive) computation for it, then emit their output. Simulations are a good example.
XML
Most XML parsers operate on whole XML documents, so if a large XML document is made up of multiple input splits, it is a challenge to parse these individually. Of course, you can process the entire XML document in one mapper (if it is not too large).
Large XML documents that are composed of a series of “records” (XML document fragments) can be broken into these records using simple string or regular-expression matching to find the start and end tags of records. This alleviates the problem when the document is split by the framework because the next start tag of a record is easy to find by simply scanning from the start of the split, just like TextInputFormat finds newline boundaries.
Hadoop comes with a class for this purpose called StreamXmlRecordReader. You can use it by setting your input format to StreamInputFor mat and setting the stream.recordreader.class property to org.apache.ha doop.streaming.mapreduce.StreamXmlRecordReader.
Binary Input
Hadoop MapReduce is not restricted to processing textual data. It has support for binary formats, too.
SequenceFileInputFormat
Hadoop SequenceFileInputFormat is an InputFormat which reads sequence files. Sequence files are binary files that stores sequences of binary key-value pairs. Sequence files are block-compressed and provide direct serialization and deserialization of several arbitrary data types (not just text). Here Key & Value both are user-defined.
SequenceFileAsTextInputFormat
Hadoop SequenceFileAsTextInputFormat is another form of Sequence FileInputFormat which converts the sequence file key values to Text objects. By calling ‘tostring()’ conversion is performed on the keys and values. This InputFormat makes sequence files suitable input for streaming.
SequenceFileAsBinaryInputFormat
Hadoop SequenceFileAsBinaryInputFormat is a SequenceFileInputFormat using which we can extract the sequence file’s keys and values as an opaque binary object.
FixedLengthInputFormat
FixedLengthInputFormat is for reading fixed-width binary records from a file, when the records are not separated by delimiters. The record size must be set via fixed lengthinputformat.record.length.
DBInputFormat
Hadoop DBInputFormat is an InputFormat that reads data from a relational database, using JDBC. As it doesn’t have portioning capabilities, so we need to careful not to swamp the database from which we are reading too many mappers. So it is best for loading relatively small datasets, perhaps for joining with large datasets from HDFS using MultipleInputs. Here Key is LongWritables while Value is DBWritables.